Understanding the Environmental Costs of AI Research
What is the Environmental Cost of AI Research?
Definition and Overview of Environmental Costs
The environmental cost of AI research refers to the ecological impact associated with the development and deployment of artificial intelligence technologies. This encompasses energy consumption, carbon emissions, resource depletion, and waste generation throughout the lifecycle of AI systems. As AI continues to proliferate across industries, understanding these costs becomes paramount for sustainable development.
Historical Context of AI Development and Environmental Impact
Historically, AI has evolved from simple algorithms to complex models requiring substantial computational power. The surge in data-driven methods, particularly deep learning, has intensified energy demands. In 2019, a study highlighted that training large AI models could emit as much carbon dioxide as five cars throughout their lifetimes. As reliance on AI grows, so does the urgency to mitigate its environmental footprint.
The Environmental Impact of Artificial Intelligence
Carbon Footprint of Machine Learning
Energy Consumption During Model Training
The energy consumption during AI model training is staggering. For instance, training a single model can consume as much energy as an average American household uses in a month. This disproportionate energy usage raises alarm bells among researchers and policymakers alike.
Lifecycle Emissions from AI Hardware
Beyond training, AI hardware also contributes significantly to carbon emissions. Manufacturing, transportation, and disposal of AI-related equipment contribute to a lifecycle carbon footprint that is often overlooked. Reports indicate that extracting the materials necessary for AI components can lead to significant environmental degradation.
Data Centers and Their Environmental Footprint
Energy Use and Source
Data centers are the backbone of AI deployment. They require vast amounts of electricity, much of which is still sourced from fossil fuels. A recent report suggests that by 2026, data centers could consume as much energy as entire countries. This dependence on non-renewable sources exacerbates the carbon footprint of AI technologies.
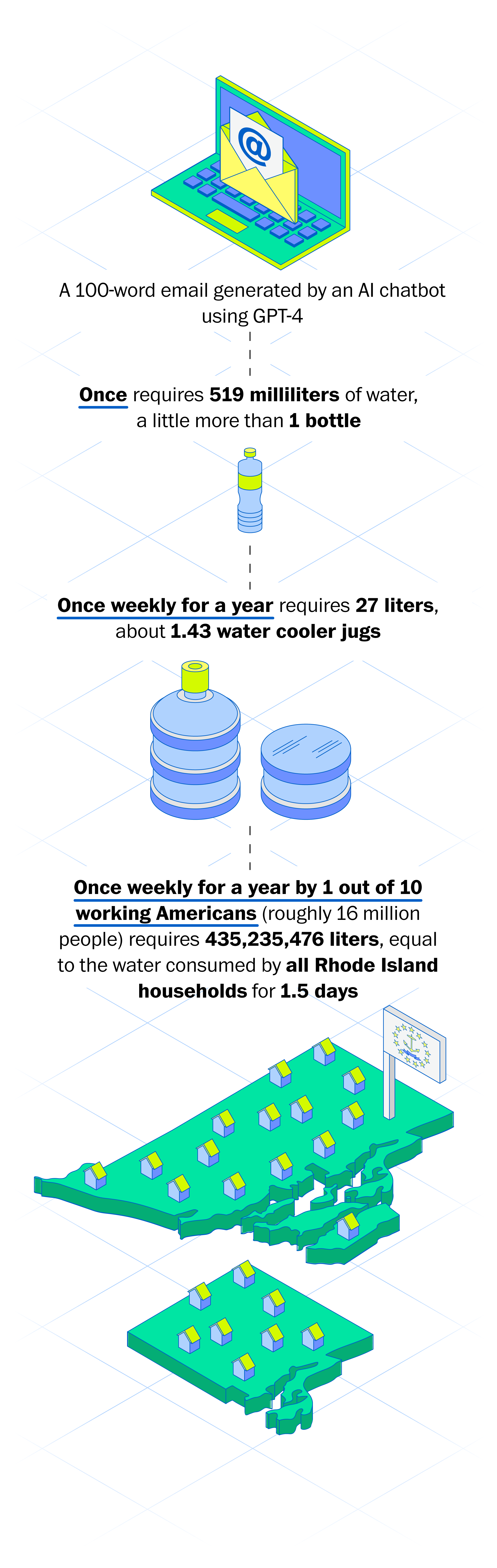
Water Consumption and Electronic Waste
In addition to energy, data centers consume water for cooling systems, which can strain local water supplies. Furthermore, the electronic waste generated from outdated hardware poses a significant environmental challenge, as it often ends up in landfills, contributing to pollution.
Sustainable AI Research Practices
Best Practices for Reducing Energy Consumption
Efficient Algorithm Design
Designing energy-efficient algorithms is crucial. By focusing on model optimization, researchers can develop algorithms that require less computational power without sacrificing performance. Techniques such as pruning and quantization can significantly lower energy requirements during training and inference.
Use of Renewable Energy in Data Centers
Transitioning data centers to renewable energy sources is a pivotal step toward reducing the environmental impact of AI. Companies like Google have committed to using 100% renewable energy in their data centers, setting a benchmark for others in the industry.
Case Studies of Sustainable AI Implementations
Analysis of Companies Leading in Green AI Initiatives
Several companies are pioneering sustainable AI practices. For example, Microsoft has implemented strategies to reduce its carbon footprint by utilizing AI to optimize energy usage in its data centers. Furthermore, numerous startups are emerging with a focus on Green AI, emphasizing the importance of sustainability in their business models.
Strategies for Reducing the Carbon Footprint of AI Development
Importance of Reporting Energy Use and Emissions
Recommendations for Research Publications
Transparency in reporting energy use and emissions associated with AI research is essential. Researchers should include energy metrics alongside traditional evaluation criteria such as accuracy and performance to foster a culture of accountability.
Transparency in AI Operations and Environmental Impact
Increased transparency from organizations about their AI operations can help stakeholders assess the environmental impact effectively. This can lead to informed decisions that prioritize sustainability in AI deployment.
Innovations in Hardware for Energy Efficiency
Advances in AI Accelerators and In-Memory Computing
Innovations in hardware, such as AI accelerators and in-memory computing, promise significant energy savings. These technologies are designed to optimize energy efficiency while maintaining high performance, addressing one of the primary concerns of AI’s environmental impact.
The Role of Photonic Chips in Reducing Energy Consumption
Photonic chips, which utilize light for data processing, present a groundbreaking opportunity to reduce energy consumption in AI. These chips can perform computations with lower energy costs compared to traditional electronic chips, potentially revolutionizing the field.
Conclusion: Balancing AI Advancements with Environmental Responsibility
Future Directions for Sustainable AI Research
The future of AI research must prioritize sustainability. This involves not only developing energy-efficient technologies but also embedding environmental considerations into the AI lifecycle. Collaborative efforts among researchers, companies, and policymakers are essential to create a framework for sustainable AI.
The Role of Policymakers and Industry Leaders in Mitigation Efforts
Policymakers must establish regulations that promote sustainable AI practices. Industry leaders should advocate for green initiatives within their organizations, fostering an ecosystem that values environmental stewardship in addition to technological advancement.
Key Takeaways
- The environmental cost of AI research includes energy consumption and carbon emissions throughout its lifecycle.
- Data centers play a significant role in the carbon footprint of AI, necessitating the use of renewable energy sources.
- Innovative hardware solutions and efficient algorithm designs can help reduce AI's energy demands.
- Transparency in reporting energy use and emissions is crucial for fostering accountability within the AI community.
- Collaboration among researchers, industry leaders, and policymakers is essential for promoting sustainable AI practices.
For further exploration on how AI is transforming environmental science, consider reading How AI is Revolutionizing Earth Systems for a Greener Future or check out the insights on Using AI to Keep Your Workplace Safe and Comfortable.